The Basics of Neural Network
Humanity has been dreaming of creating intelligent inventions since as far back as 800 BC, when ancient Greeks wrote about mechanical men created by the Gods. In the last century, technology has been progressing exponentially and has brought us to a point where that dream of self-aware robots is no longer associated with magic. The creation of Artificial Intelligence has allowed us to create amazing programs that can far surpass the abilities of any human. The key advancement in the field of artificial intelligence that allowed us to do amazing things like create chat-bots and detect malignant tumors was the discovery of neural networks. Before this, programmers created relatively simple regression models to predict and classify data. However, these models had their limitations.
What are Neural Networks
A neural network is exactly what it says in the name. It is a network of neurons that are used to process information. To create these, scientists looked at the most advanced data processing machine at the time — the brain. Our brains process information using networks of neurons. They receive an input, process it, and accordingly output electric signals to the neurons it is connected to. Using bio-mimicry, we were able to apply the architecture of our brains to further the field of artificial intelligence.
The Parts of a Neural Network
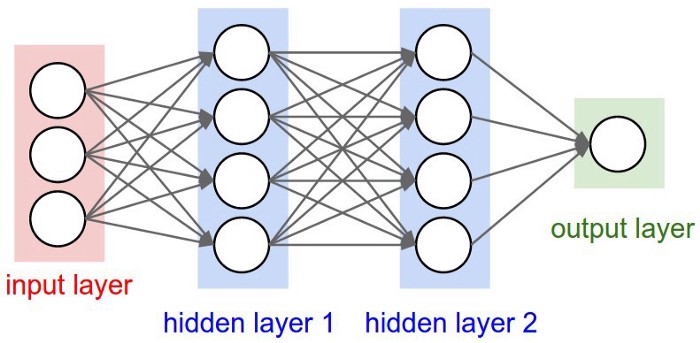
A neural network is made up of 3 main parts:
Input layer
This is literally the layer that inputs information for the neural network to process. Each circle represents 1 feature (a piece of information). This can be anything. It could be the square footage of your house for a house price prediction program, or the value of a pixel on a screen for a computer vision program
Hidden layers
These layers do all the processing for neural networks. You can have as many of these as you want. Generally speaking, the more hidden layers you have, the more accurate the neural network will be. Each layer consists of nodes that mimic our brains’ neurons. These nodes receive information from the previous layer’s nodes, multiply it by weight and then add a bias to it. Each line in the diagram represents a weight. That may sound confusing so here’s an analogy:
Let’s say I want to predict my SAT score and I have gathered two pieces of data: the number of hours I prepared, and the breakfast I had on the day of the test. These would go into the input layer. It’s quite obvious that the hours I spend preparing will have a much greater impact on my mark than my breakfast (or everyone would ace the SAT). Because of this, the hours I spend studying receive a significantly larger weight value to be multiplied by. Now, granted, there’s a chance that your breakfast may very slightly impact your score, so it will receive a much lower weight value.
Output Layer
This layer simply brings together the information from the last hidden layer of the network to output all the information you need from the program.
This brings us to end of this session.
Tagged withNote : These are the random articles took from Medium